Data Analytics Project
Improving Customer Retention with Predictive Analytics
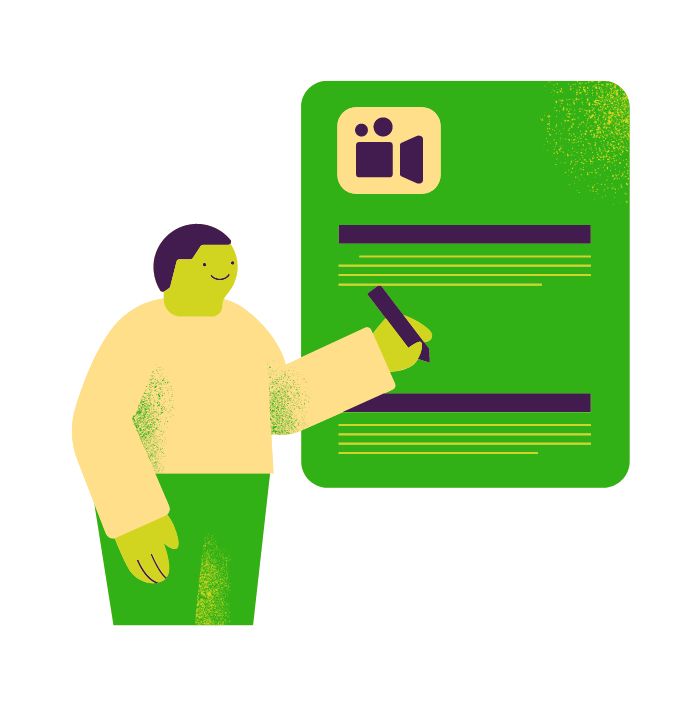
Current State
A subscription-based e-commerce company was facing a high churn rate, impacting revenue and growth. The company lacked insights into customer behavior and the factors leading to churn, making it difficult to implement effective retention strategies.
Research
Extensive research was conducted to understand customer behavior and identify the drivers of churn:
- Analyzing historical customer data, including purchase history, engagement metrics, and support interactions.
- Conducting surveys and feedback sessions with customers to gather qualitative insights.
- Reviewing industry benchmarks and best practices for customer retention.
- Identifying key metrics and potential predictors of churn.
Approach
The approach was structured in the following phases:
- Data Collection: Gathering and consolidating data from various sources such as CRM, website analytics, and customer support systems.
- Exploratory Data Analysis (EDA): Conducting EDA to identify patterns and correlations in the data.
- Predictive Modeling: Developing machine learning models to predict customer churn based on historical data.
- Segmentation: Segmenting customers based on their likelihood to churn and other key attributes.
- Strategy Development: Creating targeted retention strategies for different customer segments based on model predictions.
Solution
A comprehensive data analytics solution was implemented, focusing on predictive modeling and actionable insights. Key components included:
- Data Pipeline: Building a robust data pipeline to collect, process, and store data from multiple sources.
- Predictive Model: Developing and training machine learning models (e.g., logistic regression, random forest) to predict churn probability.
- Customer Segmentation: Segmenting customers into risk categories (e.g., high, medium, low) based on churn probability scores.
- Dashboard: Creating an interactive dashboard to visualize churn predictions, segment insights, and key retention metrics.
- Retention Strategies: Designing personalized retention strategies such as targeted offers, personalized communication, and proactive customer support.
Impact
The implementation of the data analytics solution significantly improved customer retention metrics:
- Reduced Churn Rate: The predictive model enabled proactive intervention, reducing the churn rate by 15%.
- Increased Customer Lifetime Value (CLV): Targeted retention strategies led to a 20% increase in average CLV.
- Enhanced Customer Insights: Detailed segmentation provided deeper insights into customer behavior and preferences.
- Improved Decision-Making: The interactive dashboard empowered the marketing and support teams with real-time data and actionable insights.
- Revenue Growth: Improved retention contributed to a 25% increase in overall revenue within six months.
This project provided the company with the tools and insights needed to effectively manage customer churn, enhance retention, and drive sustainable growth.